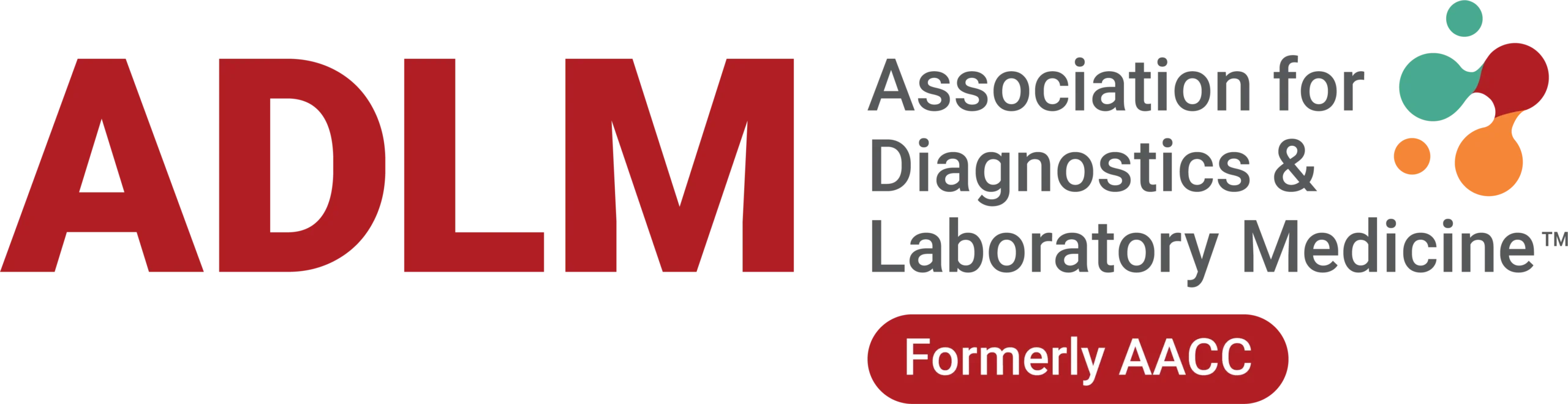
Meet Dendi at ADLM Clinical Lab Expo, July 30 – August 1 in Chicago!
Schedule to meet at our booth for a free expo pass! See event details here >
Executive War College 2024
Post-Event Recap (Part 1) – All Eyes on AI in Diagnostic Labs
May 2024 – The annual 2024 Executive War College (EWC) conference on diagnostics, clinical laboratory, and pathology management commenced in “The Big Easy” bringing together 1,034 lab professionals – breaking the previous record of 953 attendees. In addition to being a fantastic networking event, EWC also assembles a schedule full of insightful seminars and workshops led by various diagnostics industry leaders.
This would mark Dendi’s fourth appearance at EWC – this year as a proud sponsor. We convened with various lab professionals including our strategic partners, long-time customers, and many newly made acquaintances to connect and share ideas about how to advance diagnostics together. If you missed out, this recap can help you get caught up until the next year. We’ll highlight the trending topics from EWC and share some of our insights.
The recap is partitioned into two parts: the current part covering AI in diagnostic labs and part 2 covering the latest updates to the regulatory environment and billing challenges (to be posted on our website).
Examining Clinical AI Applications from All Angles at EWC
With all the recent buzz surrounding generative AI like ChatGPT, it was no surprise that artificial intelligence was a hot topic this year, with over a dozen scheduled sessions focused on different aspects of AI applications in healthcare. These are our notes and thoughts from selected sessions.
Content Outline:
(jump to content)
- The roadblocks facing adoption of AI in diagnostic labs – What the US could learn from the UK in order to adopt AI and other innovative technology to advance healthcare faster.
- Five business models of AI applications – Examples of the innovative ways healthcare companies are utilizing AI today.
- Taking advantage of AI in diagnostic labs today – Considerations for building an AI strategy.
- Insights and takeaways from Dendi – Looking beyond the hype and identifying feasible first steps.
Exploring the Intersections and Opportunities with Big Data, Precision Medicine, and AI in Diagnostic Labs and Clinical Care
In the fireside chat session, Michael Simpson (President & CEO, Clinisys), Leo Grady (CEO & Co-Founder, Jona), Joseph Mossel (CEO & Co-Founder, Ibex Medical Analytics), and Ajit Singh (Managing Director, Artiman Ventures) answered questions from the audience and shared their views about the opportunities and challenges ahead for AI adoption in the healthcare industry.
Ajit Singh discussed how perceived value affects AI adoption. He compared it to consumer goods, stating that brand perception and understanding the tangible benefits of AI are crucial for its acceptance.
To explain the barriers to AI adoption in the US, Leo Grady presented a comparison between how the US and the UK healthcare systems approach adopting new technology:
“In the US, you have to collect data and then figure out how much to pay for it. But no one wants to use the technology and collect data until it’s getting paid. Whereas in the UK, they flip that equation.”
Grady explained that the UK has implemented programs (such as the AI Diagnostic Fund and the Investigational Technology Program) that provide revenue incentives to kickstart the adoption of new technology. It can then evaluate the resulting value created before rolling it out on a larger scale. This creates a willingness to accept more risk as a health system and enable faster adoption of technology.
Michael Simpson expanded upon the comparison between the US and UK health systems and pointed out that the main difference is cultural.
“The way we think [in the US] is quarter by quarter. We’re playing the short game; they’re playing the long game. When we look at digital pathology, and why [the UK] is so much faster at adoption, it’s because they are looking three years down the road.”
He revealed that in discussions with the UK’s National Health Service, they found a concerning trend: the declining number of new pathologists entering the workforce and the existing shortage would eventually be unsustainable. To address this, the NHS is proactively investing in solutions now to support pathologists in meeting future healthcare demands.
The chat concluded on an optimistic note, encouraging the audience to view AI as a transformative tool that can enhance medical research, diagnostics, and patient care, beyond just automating tasks. AI should instead be viewed as “augmented intelligence” that would serve to assist humans, not take them out of the picture.
But there is a long road ahead. Before AI can be utilized, the US health system needs to embrace industry-wide collaboration in order to determine the value that AI technology can bring towards the advancement of healthcare. Until then, even the most ambitious players will have trouble gaining any traction.
Applications of AI in Diagnostic Labs and Clinical Practices
Leo Grady, CEO of Jona, presented insights into AI’s diverse potential in healthcare, giving examples of how it’s being integrated today including an overview of Jona’s gut microbiome analysis solution. Key points from this session:
- Financial Viability: AI adoption hinges on robust, unbiased, and clinically validated systems that can also demonstrate clear financial returns, but will first require serious financial investment to get to that point.
- Five business models for AI and examples:
- Vertical integration: GE HealthCare’s strategy is to integrate AI into their precision care frameworks and medical devices to offer comprehensive, data-driven solutions to identify potential theranostics candidates.
- Efficiency and value-based care: Atropos Health’s generative AI platform produces high-quality, personalized real-world evidence to enhance value-based care clinical decision-making.
- Sell to pharmaceutical companies: GlaxoSmithKlein partners with PathAI to bring digital pathology AI to cancer drug development
- Direct to Consumer (cash payment models): Levels Health, Prenuvo, Ouro Ring
- Perceived value must exceed its cost: Adoption relies heavily on AI applications being perceived as valuable. Factors such as data quality, brand strength, and comparative value play critical roles.
Slide: Five business models for AI in healthcare (credit: Jona)
How Labs Can Get Value From AI NOW
In this session Brad Bostic, Chairman & CEO of hc1 Insights, discussed the impact and strategic implementation of artificial intelligence (AI) and machine learning (ML) in laboratory settings, as well as first steps that labs can take to prepare for AI applications.
- AI Transformation in Healthcare: AI is transforming operational processes by enabling large-scale data analysis that humans cannot match, specifically in optimizing laboratory operations and diagnostic decisions.
- Examples and Applications:
- AI-driven workforce optimization to align staff levels with actual needs.
- Predictive models for health conditions like cognitive decline, leveraging routine lab data.
- hc1 uses AI to manage underutilization and overutilization of tests, aiming to streamline operations and enhance diagnostic accuracy.
- AI’s Potential and Limitations: While AI promises significant advancements, it inherently lacks human qualities such as emotional intelligence, emphasizing that AI will augment but not replace human roles in healthcare.
- Impact on Pathology: AI offers an opportunity to reposition pathology and laboratory services more centrally within the healthcare continuum, similar to how radiology is currently viewed.
Questions from the audience led to insightful discussions:
- Leveraging AI in clinical decision support while keeping its limitations and risks in mind: Questions were raised about the regulatory and practical implications of AI in clinical settings, especially when AI systems provide diagnostic support. Bostic emphasized that AI should be viewed as a tool to support, not substitute, professional medical judgment. He suggested that AI be thought of as “augmented intelligence” to help illustrate this point. There’s importance in incorporating human oversight in AI applications to counteract biases and incorrect outputs due to data limitations. The group advocated for ‘human-in-the-loop’ systems to ensure AI tools are used as aides, not replacements.
- Financial investment considerations for AI implementation: In response to an inquiry on how small labs can adopt AI without significant investment. Bostic highlighted the accessibility of modern cloud-based tools that allow even small-scale operations to benefit from AI with minimal initial investment.
- Data quality is more important than data quantity for AI model training. The necessity of substantial, high-quality data to train models was underscored, alongside the continuous refinement of these models based on operational feedback.
Slide: Steps for implementing the infrastructure for AI applications (credit: hc1 Insights)
The consensus was that while AI in diagnostics is advancing rapidly, the technology’s integration must be handled carefully to enhance healthcare delivery without compromising the human elements crucial to patient care.
Artificial Intelligence: Regulatory and other Legal Issues. A Primer for Clinical Laboratories
With new transformative technology like AI, comes regulatory considerations. Roger D. Klein MD, JD led a session to describe the legal implications of using AI in clinical laboratories.
- Legal Responsibility and Liability:
- Key Stakeholders: Treating physicians, pathologists, lab directors, institutions, and software developers each have potential liability in the deployment and use of AI.
- Issues of Accountability: Errors or failures of AI in clinical settings might allocate responsibility among these stakeholders, which could involve complex legal battles over malpractice or negligence.
- Contractual Obligations and Licensing:
- Risk Allocation: Essential for contracts to explicitly address which party bears the risk for AI failures, with indemnification clauses to protect against liabilities arising from AI’s use.
- Data Usage and Ownership: Contracts must clearly define terms regarding the ownership, use, and transferability of data used by AI, which is crucial for maintaining compliance with privacy laws and protecting proprietary information.
- Regulatory Compliance:
- FDA Oversight: AI applications that qualify as Software as a Medical Device (SaMD) come under FDA scrutiny. The FDA provides a framework to ensure that AI products used in healthcare are safe, effective, and secure.
- FTC and Privacy: AI must comply with Federal Trade Commission (FTC) guidelines to avoid unfair or deceptive practices. This includes adherence to privacy standards and the accurate representation of AI capabilities and limitations.
- Intellectual Property (IP) Concerns:
- IP Ownership: Clarifies that AI-generated outputs (like data or reports) can raise questions about authorship and ownership, impacting patents, copyrights, and trade secrets.
- Non-Infringement: Contracts should ensure that AI systems do not infringe on existing intellectual property rights, which could lead to legal disputes.
- Product Liability:
- Application Errors: If AI tools malfunction, leading to patient harm or data breaches, liability could extend to manufacturers and laboratories.
- Novel AI Issues: Product liability law needs to adapt to address unique challenges posed by AI, such as autonomous decision-making and continuous learning capabilities that may alter the tool’s behavior over time.
- Antitrust Implications:
- Competitive Concerns: AI’s ability to process vast amounts of data and respond to market conditions might inadvertently lead to anti-competitive practices such as price-fixing or market manipulation.
- Regulatory Surveillance: Ensuring AI systems do not facilitate or engage in anti-competitive agreements is essential for compliance with antitrust laws.
These points highlight some of the legal considerations of implementing AI in clinical laboratories, emphasizing the need for careful planning, clear contractual agreements, and adherence to a broad spectrum of regulatory requirements to mitigate risks associated with AI deployment in healthcare.
Dendi’s Takeaways: Preparing Your Lab for AI
Why Your Lab’s AI Strategy Might Be Premature
While there is immense optimism for AI’s potential for healthcare, a recurring theme during this year’s EWC was the necessity for industry-wide collaboration and clear value demonstration before AI applications can gain traction in the clinical laboratory space. Speakers emphasized that without strong incentives and evidence-based benefits, stakeholders are unlikely to embrace AI due to concerns over significant operational disruptions, potential threats to human expertise, and the elimination of jobs for doctors, nurses, and other providers.
Moreover, despite the maturity of generative AI, there are still significant challenges, such as the tendency for large language models (LLMs) to “hallucinate” or generate inaccurate information. This necessitates a continued human role in validating AI-generated content.
Start with What You Can Control
For labs eager to explore AI, it’s crucial to first evaluate for tech-readiness by successfully adopting tech-driven automation on a smaller scale. For instance, during EWC, we were approached by several labs asking, “Does your LIS have AI?” without a clear understanding of specific goals for “the AI solution” to address. It’s essential to identify and focus on specific desired outcomes – feasible solutions may already exist that have nothing to do with AI.
We spoke with a lab who shared their struggles with performance and scalability issues due to their current LIS’s cloud-hosted database. They faced long query times due to the sheer volume of data (over 50,000 tests per month). This highlights a common problem: a legacy system on outdated IT infrastructure becomes especially problematic with large datasets and complex queries. Some labs still run manual SQL queries on server-hosted or cloud-enabled databases that are not easily scalable to generate frequent operational reports. Many more lab systems are capable of even less than that.
Since this is the current reality, the lab industry would be getting ahead of itself to believe that it is anywhere near ready for clinical applications of LLMs, training AI models, and generative AI using real-time data.
Questions to Assess AI-Readiness
When considering an AI strategy, labs should first evaluate their current IT infrastructure with questions like:
Is the lab system’s data accessible externally?
Is the data structured and clean enough to be used for analytics?
Can the usable data be easily extracted for analysis?
Is your current system capable of modern integrations with cloud-based tools and services?
If the answer to any of these questions is no, the primary priority should be to modernize infrastructure. This will not only address immediate needs but also prepare the lab for next-gen solutions, whether AI or otherwise.
Focus on High-Value Solutions First
While the potential of AI in diagnostics is immense, it’s important for labs to temper their expectations and not rush into AI adoption. It’s likely that we’re currently in a “peak hype phase”, and practical, regulatory-compliant AI applications for high-risk clinical settings are still in early development. Instead, labs should focus on modernizing their IT infrastructure and focus on the immediate issues at hand which are largely-related to healthcare systems integrations to improve the user experience and operational efficiency. Only when labs are able to effectively manage and exchange data, can they ensure they are prepared to leverage AI when it becomes viable.
A Modern LIS Lays the Foundation for AI and other Advancements
From its inception, Dendi LIS was designed as a future-proof solution to support the current needs of labs while enabling them to scale with future innovations via modern integration capabilities. Many issues laboratories face stem from outdated IT infrastructure with insufficient capabilities. Addressing this fundamental issue is the first step to advancing clinical diagnostics.
Labs that choose an LIS partner that can facilitate a successful system transition, are positioning themselves to capitalize on AI and other advanced technologies as they mature, ensuring that they stay ahead in the ever-evolving field of clinical diagnostics. To discover Dendi’s all-in-one LIS platform, visit www.dendisoftware.com.
We extend our deepest gratitude to the Dark Intelligence Group for organizing another outstanding Executive War College and to all the attendees and speakers who shared their invaluable insights and experiences. Looking forward to next year!